
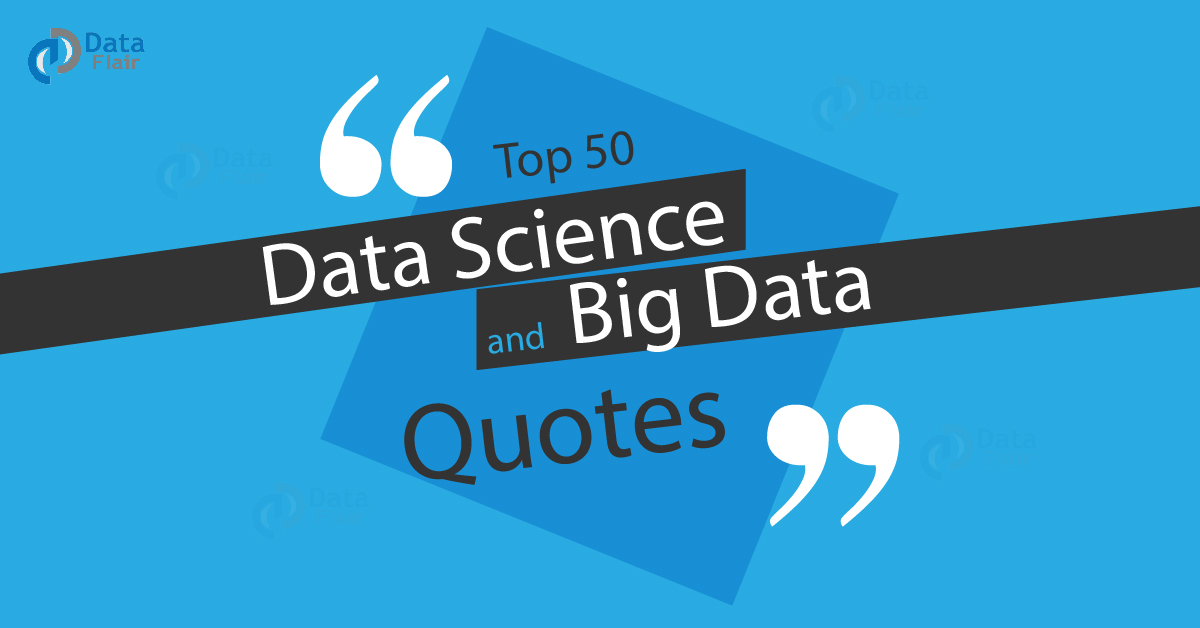
We mostly use databases with a Database Management System (DBMS), like PostgreSQL or MySQL. You then use those common traits as a guide for what category the new item might have.Īs simply as possible, this is a storage space for data. You take a set of data where every item already has a category and look at common traits between each item. It deals with categorizing a data point based on its similarity to other data points. The problems we must address with big data are categorized by the 4 V’s: volume, variety, veracity, and velocity.Ĭlassification is a supervised machine learning problem. It’s more helpful to read it as, “so much data that you need to take careful steps to avoid week-long script runtimes.” Big data is more about strategies and tools that help computers do complex analysis of very large (read: 1+ TB) data sets. You can think of the back end as the frame, the plumbing, and the wiring of an apartment.īig data is a term that suffers from being too broad to be useful. This includes databases, servers, authentication procedures, and much more.
#Scientific quotes database code#
The back end is all of the code and technology that works behind the scenes to populate the front end with useful information. This can be as easy as finding and removing every comma in a paragraph, or as complex as building an equation that predicts how many home runs a baseball player will hit in 2018.

While you probably won’t have to work with every concept mentioned here, knowing what the terms mean will help when reading articles or discussing topics with fellow data lovers.Īn algorithm is a set of instructions we give a computer so it can take values and manipulate them into a usable form. These are some baseline concepts that are helpful to grasp when getting started in data science. We hope it will serve as your handy quick reference whenever you’re working on a project, or reading an article and find you can’t quite remember what “ETL” means. To help those new to the field stay on top of industry jargon and terminology, we’ve put together this glossary of data science terms. Even the term “data science” can be somewhat nebulous, and as the field gains popularity it seems to lose definition. Getting started in data science can be overwhelming, especially when you consider the variety of concepts and techniques a data scienctist needs to master in order to do her job effectively.
